⚔️ OpenAI vs. Open AI + 🐝 Swarm for Multi-Agent AI
Plus, Tesla's AI-first robotics, Meta's "thinking" model TPO, and a game-changing AI simulation!
Welcome to this week in AI.
This week's news: OpenAI goes to war with... Open AI (yes, you read that right), Amazon reshapes online shopping with AI-powered guides, and Tesla’s AI-first approach leads the way in robotics.
Plus, explore OpenAI's experimental Swarm for multi-agent systems, Meta’s new "thinking" model TPO, and DIAMOND's breakthrough in game simulation.
Let’s get caught up!
Don’t feel like reading? Listen instead.
Don't Miss Out
Join me at the QLD AI Meetup on October 30th in Brisbane to discuss redefining UX for AI, plus get my upcoming Ultimate Guide For Designing AI Systems.
OpenAI Goes to War With Open AI
OpenAI is embroiled in a trademark dispute with Guy Ravine, who owns the 'Open AI' (with a space) trademark.
Ravine registered the domain open.ai in March 2015 and claims he conceived and pitched the idea for the initiative to major tech leaders before OpenAI's founders.
The legal conflict began when OpenAI noticed Ravine's domain redirecting to their website, raising concerns about potential association or affiliation.
Why It Matters
This case underscores the complexities of intellectual property in the rapidly evolving AI sector, particularly as many AI startups use existing business names combined with the .ai domain extension for their online presence.
Interestingly, the .ai domain isn’t tied to AI at all—it’s actually the Internet country code top-level domain for Anguilla, a British Overseas Territory in the Caribbean (similar to .au for Australia).
Despite this, the .ai extension has become highly popular among companies and projects in the artificial intelligence industry.
This trademark dispute serves as a reminder for companies in the AI space to secure intellectual property early and carefully consider naming and branding strategies to avoid similar conflicts.
📰 Article by Bloomberg (Paywalled)
📝 Dry legal notes on the matter
AI-Powered Amazon Guides: Reshaping Online Shopping
Amazon has launched a new AI-powered feature called "Shopping Guides" for its US mobile platforms, covering over 100 product types, including TVs, headphones, shoes, and skincare.
This innovative tool aims to streamline the product discovery process by providing tailored information and recommendations to users.
The AI guides offer a more visual and intuitive approach to filtering products, moving away from traditional tick-box menus.
They present "factors to consider" that break down products into specific categories, helping shoppers understand various features and make more informed purchase decisions.
Why It Matters
This update to Amazon's shopping experience is significant for consumers who often face decision fatigue when confronted with countless options online.
By leveraging AI to provide educational content and customer insights, Amazon is making it easier for shoppers to find products that truly meet their needs.
The feature's ability to automatically appear for relevant autocomplete search suggestions and its integration with the "Keep Shopping For" cards demonstrates Amazon's commitment to creating a more personalised and efficient shopping journey.
For those less familiar with specific product features, this tool offers a way to quickly get up to speed without extensive research.
As e-commerce continues to grow, innovations like AI Shopping Guides are set to enhance the online shopping experience, potentially saving consumers time and leading to more satisfying purchases.
OpenAI’s Swarm: Advancing Autonomous AI with Scalable Multi-Agent Systems
OpenAI’s swarm is an experimental library for building multi-agent systems, using its Chat Completions API to enable stateless, dynamic agent orchestration.
It supports direct Python function calls, allowing agents to handle tasks and switch responsibilities through a handoff mechanism.
Swarm’s design focuses on flexibility and scalability, making it easy for developers to manage complex agent interactions in real time.
This tool also aligns with OpenAI's AGI roadmap, particularly Level 3: Agents, where AI systems can take independent actions.
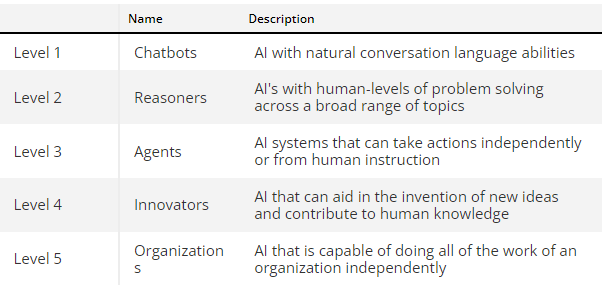
Swarm contributes to advancing autonomous AI, laying the groundwork for agents capable of performing tasks without constant human input.
Why it Matters
Swarm enables the creation of scalable, autonomous AI systems, offering developers control over multi-agent interactions.
By supporting Python function calls and dynamic task switching, it simplifies agent management and expands AI’s capabilities.
Swarm is a crucial step toward autonomous agents in OpenAI’s roadmap to AGI, pushing AI closer to independent, real-world applications.
AI Beyond the Wheel: Andrej Karpathy on Tesla and Robotics
In this week’s No Priors podcast, Andrej Karpathy, former Tesla Autopilot leader and founding member of OpenAI, discussed the evolution of self-driving cars and Tesla’s AI-first approach.
He explained how Tesla's focus on software, using neural networks and camera-based vision systems, allowed it to scale more efficiently than Waymo, which relies on expensive hardware like LiDAR.
Karpathy emphasised that Tesla’s ultimate goal is to achieve end-to-end deep learning, where AI takes over almost all driving tasks.
He also covered Tesla’s humanoid robot, Optimus, highlighting how AI systems developed for cars are being repurposed for robotics, especially for tasks like material handling in factories.
Why it Matters
Tesla’s AI-first, software-based approach has positioned it to lead the self-driving car market by minimising dependence on costly hardware.
This scalable solution could accelerate the deployment of autonomous vehicles globally, reshaping the future of transport.
Karpathy’s insights into Tesla’s robotics programme suggest that similar AI systems will drive automation in industries like manufacturing, enhancing efficiency across sectors.
DIAMOND: Advancing AI Simulation with Diffusion Models and Minimal Data
Researchers from the University of Geneva, University of Edinburgh, and Microsoft have developed DIAMOND, an AI model capable of simulating environments, including a playable version of Counter-Strike (CS) at 10 frames per second.
Using a diffusion-based approach, the model predicts the next game frame based on previous ones and user actions.
Remarkably, DIAMOND was trained using only 87 hours of CS gameplay, significantly less than the typical data used in similar AI models, such as Google’s DOOM simulation.
The model achieved impressive results, performing 46% better than human-level agents on the Atari 100k benchmark—a new state-of-the-art (SOTA) performance for world model-trained agents.
This AI-driven simulation not only mimics player interactions and game mechanics but also showcases improvements in visual detail modeling, crucial for reinforcement learning performance.
DIAMOND demonstrates the growing potential for diffusion models in game simulation and broader applications, such as robotics and autonomous systems.
Why it Matters
DIAMOND's breakthrough in generating interactive, physics-based game environments using minimal data offers a glimpse into the future of AI training.
The model’s ability to operate with only 87 hours of gameplay yet still outperform human benchmarks underscores its efficiency and the potential for cost-effective, scalable AI systems.
These advancements are relevant beyond gaming, with applications in robotics and autonomous systems that can benefit from AI-trained simulations in virtual environments.
As AI continues to refine its methods for processing and predicting complex, real-world tasks, DIAMOND highlights how diffusion models could shape the future of reinforcement learning and interactive technologies.
📝 Project landing page (try it yourself)
AI Learns to Think: Meta’s TPO and Its Implications for Non-Reasoning Tasks
Meta researchers have introduced Thought Preference Optimization (TPO), a novel method for training large language models to "think" before responding to user instructions, even in non-reasoning tasks.
Unlike traditional models, which immediately generate responses, TPO prompts AI to generate internal thoughts first, similar to how humans consider before speaking.
This method has shown improved performance in tasks such as creative writing, marketing, and general instruction-following, outperforming other models in benchmarks like AlpacaEval with a win rate of 52.5%. However, it struggles in specialised tasks like mathematics, where its performance falls short of base models.
TPO operates by generating thought steps and evaluating only the final answers, allowing the model to optimise its responses through implicit learning.
This builds on previous research from OpenAI, notably their o1 model, which also explores reasoning capabilities but in a more task-specific manner. The introduction of TPO could push AI's utility further into creative, flexible, and less structured domains.
Why it Matters
TPO represents an important development for AI's application in creative and non-reasoning tasks, such as marketing, common sense, and health.
By enabling models to "think" before answering, this method broadens AI's potential beyond traditional logic-based tasks, offering businesses and creative professionals more advanced AI tools.
The technology could enhance the quality of AI-generated content, making it a valuable asset for industries that rely on creativity and nuanced decision-making.
While challenges remain for more specialised areas like mathematics, the advancement opens up new possibilities for AI in diverse fields.
Cease-and-Desist: NYT Takes Aim at Perplexity’s Use of Its Content
The New York Times (NYT) has issued a cease-and-desist notice to the AI search startup Perplexity, accusing the company of using its content for AI-generated summaries without permission, which NYT claims violates copyright law.
Despite Perplexity's previous assurances to stop crawling NYT's content, its articles continue to appear on the platform.
The startup maintains that it does not scrape content for building AI models but instead indexes web pages for factual citations in responses to user queries.
Perplexity has until October 30 to respond to the notice, while NYT has previously taken legal action against other AI companies like OpenAI for similar infractions.
Why it Matters
This conflict highlights the growing tension between traditional media outlets and AI platforms, as publishers fear that AI-generated summaries may reduce their traffic and revenue.
The legal battles over copyright infringement in AI are becoming more frequent, raising important questions about how AI can ethically and legally use content.
As AI search tools like Perplexity continue to evolve, the publishing industry is grappling with the potential impact on their business models, making this a significant issue for both tech and media sectors.